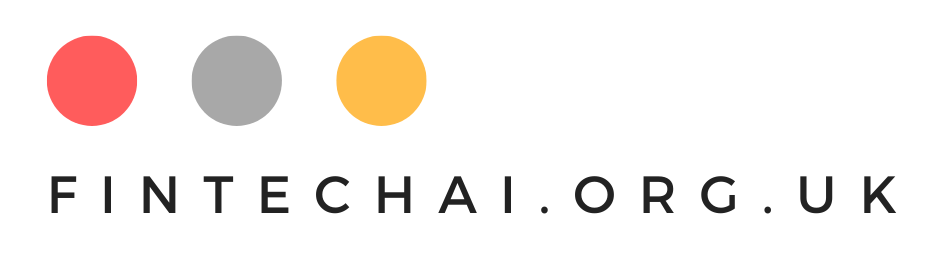
Infographics of Recent Publications
Estimation Based on Nearest Neighbor Matching: From Density Ratio to Average Treatment Effect
Econometrica, 2023
Lin, Zhexiao; Ding, Peng; Han, Fang
Nearest neighbor (NN) matching is widely used in observational studies for causal effects. Abadie and Imbens (2006) provided the first large-sample analysis of NN matching. Their theory focuses on the case with the number of NNs, M fixed. We reveal something new out of their study and show that once allowing M to diverge with the sample size an intrinsic statistic in their analysis constitutes a consistent estimator of the density ratio with regard to covariates across the treated and control groups. Consequently, with a diverging M, the NN matching with Abadie and Imbens' (2011) bias correction yields a doubly robust estimator of the average treatment effect and is semiparametrically efficient if the density functions are sufficiently smooth and the outcome model is consistently estimated. It can thus be viewed as a precursor of the double machine learning estimators.
The Virtue of Complexity in Return Prediction
Journal of Finance, 2024
Kelly, Bryan; Malamud, Semyon; Zhou, Kangying
Much of the extant literature predicts market returns with "simple" models that use only a few parameters. Contrary to conventional wisdom, we theoretically prove that simple models severely understate return predictability compared to "complex" models in which the number of parameters exceeds the number of observations. We empirically document the virtue of complexity in U.S. equity market return prediction. Our findings establish the rationale for modeling expected returns through machine learning.
Lender Automation and Racial Disparities in Credit Access
Journal of Finance, 2024
Howell, Sabrina T.; Kuchler, Theresa; Snitkof, David; Stroebel, Johannes; Wong, Jun
Process automation reduces racial disparities in credit access by enabling smaller loans, broadening banks' geographic reach, and removing human biases from decision making. We document these findings in the context of the Paycheck Protection Program (PPP), where private lenders faced no credit risk but decided which firms to serve. Black-owned firms obtained PPP loans primarily from automated fintech lenders, especially in areas with high racial animus. After traditional banks automated their loan processing procedures, their PPP lending to Black-owned firms increased. Our findings cannot be fully explained by racial differences in loan application behaviors, preexisting banking relationships, firm performance, or fraud rates.
Charting by Machines
Journal of Financial Economics, 2024
Murray, Scott; Xia, Yusen; Xiao, Houping
We test the efficient market hypothesis by using machine learning to forecast stock returns from historical performance. These forecasts strongly predict the cross-section of future stock returns. The predictive power holds in most subperiods and is strong among the largest 500 stocks. The forecasting function has important nonlinearities and interactions, is remarkably stable through time, and captures effects distinct from momentum, reversal, and extant technical signals. These findings question the efficient market hypothesis and indicate that technical analysis and charting have merit. We also demonstrate that machine learning models that perform well in optimization continue to perform well out-of-sample.
Machine Learning as a Tool for Hypothesis Generation
Quarterly Journal of Economics, 2024
Ludwig, Jens; Mullainathan, Sendhil
While hypothesis testing is a highly formalized activity, hypothesis generation remains largely informal. We propose a systematic procedure to generate novel hypotheses about human behavior, which uses the capacity of machine learning algorithms to notice patterns people might not. We illustrate the procedure with a concrete application: judge decisions about whom to jail. We begin with a striking fact: the defendant's face alone matters greatly for the judge's jailing decision. In fact, an algorithm given only the pixels in the defendant's mug shot accounts for up to half of the predictable variation. We develop a procedure that allows human subjects to interact with this black-box algorithm to produce hypotheses about what in the face influences judge decisions. The procedure generates hypotheses that are both interpretable and novel: they are not explained by demographics (e.g., race) or existing psychology research, nor are they already known (even if tacitly) to people or experts. Though these results are specific, our procedure is general. It provides a way to produce novel, interpretable hypotheses from any high-dimensional data set (e.g., cell phones, satellites, online behavior, news headlines, corporate filings, and high-frequency time series). A central tenet of our article is that hypothesis generation is a valuable activity, and we hope this encourages future work in this largely "prescientific" stage of science.