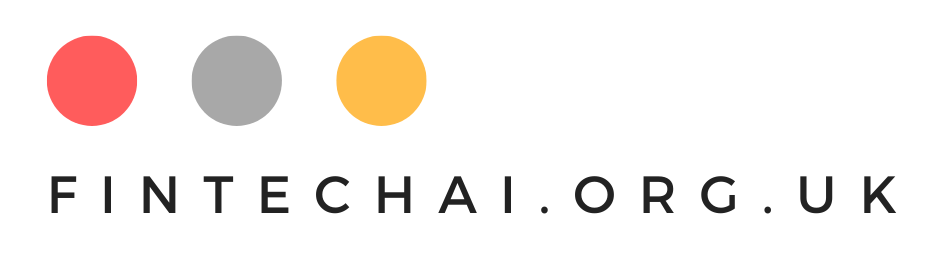
Infographics of Publications in Review of Financial Studies
The Partisanship of Financial Regulators
Review of Financial Studies, 2023
Engelberg, Joseph; Henriksson, Matthew; Manela, Asaf; Williams, Jared
We analyze the partisanship of Commissioners at the SEC and Governors at the Federal Reserve Board. Using recent advances in machine learning, we identify partisan phrases in Congress, such as "red tape" and "climate change," and observe their usage among regulators. Although the Fed has remained relatively nonpartisan throughout our sample period (1930-2019), we find that partisanship among SEC Commissioners rose to an all-time high during the 2010-2019 period, driven by more-partisan Commissioners replacing less-partisan ones. Partisanship at the SEC appears in both the language of new SEC rules and the voting behavior of SEC Commissioners.
Option Return Predictability with Machine Learning and Big Data
Review of Financial Studies, 2023
Bali, Turan G.; Beckmeyer, Heiner; Morke, Mathis; Weigert, Florian
Drawing upon more than 12 million observations over the period from 1996 to 2020, we find that allowing for nonlinearities significantly increases the out-of-sample performance of option and stock characteristics in predicting future option returns. The nonlinear machine learning models generate statistically and economically sizable profits in the long-short portfolios of equity options even after accounting for transaction costs. Although option-based characteristics are the most important standalone predictors, stock-based measures offer substantial incremental predictive power when considered alongside option-based characteristics. Finally, we provide compelling evidence that option return predictability is driven by informational frictions and option mispricing. Authors have furnished an Internet Appendix, which is available on the Oxford University Press Web site next to the link to the final published paper online.
Man versus Machine Learning: The Term Structure of Earnings Expectations and Conditional Biases
Review of Financial Studies, 2023
van Binsbergen, Jules H.; Han, Xiao; Lopez-Lira, Alejandro
We introduce a real-time measure of conditional biases to firms' earnings forecasts. The measure is defined as the difference between analysts' expectations and a statistically optimal unbiased machine-learning benchmark. Analysts' conditional expectations are, on average, biased upward, a bias that increases in the forecast horizon. These biases are associated with negative cross-sectional return predictability, and the short legs of many anomalies contain firms with excessively optimistic earnings forecasts. Further, managers of companies with the greatest upward-biased earnings forecasts are more likely to issue stocks. Commonly used linear earnings models do not work out-of-sample and are inferior to those analysts provide.
Credit Building or Credit Crumbling? A Credit Builder Loan's Effects on Consumer Behavior and Market Efficiency in the United States
Review of Financial Studies, 2023
Burke, Jeremy; Jamison, Julian; Karlan, Dean; Mihaly, Kata; Zinman, Jonathan
A randomized encouragement design yields null average effects of a credit builder loan (CBL) on consumer credit scores. But machine learning algorithms indicate the nulls are due to stark, offsetting treatment effects depending on baseline installment credit activity. Delinquency on preexisting loan obligations drives the negative effects, suggesting that adding a CBL overextends some consumers and generates negative externalities on other lenders. More favorably for the market, CBL take-up generates positive selection on score improvements. Simple changes to CBL practice, particularly to provider screening and credit bureau reporting, could ameliorate the negative effects for consumers and the market.
When FinTech Competes for Payment Flows
Review of Financial Studies, 2022
Parlour, Christine A.; Rajan, Uday; Zhu, Haoxiang
We study the impact of FinTech competition in payment services when a monopolist bank uses payment data to learn about consumers' credit quality. Competition from FinTech payment providers disrupts this information spillover. The bank's price for payment services and its loan offers are affected. FinTech competition promotes financial inclusion, may hurt consumers with a strong bank preference, and has an ambiguous effect on the loan market. Both FinTech data sales and consumer data portability increase bank lending, but the effects on consumer welfare are ambiguous. Under mild conditions, consumer welfare is higher under data sales than with data portability.
Small Bank Lending in the Era of Fintech and Shadow Banks: A Sideshow?
Review of Financial Studies, 2022
Begley, Taylor A.; Srinivasan, Kandarp
Amid the emerging dominance of nonbanks, small banks use key financing advantages to persist in the mortgage market. We provide evidence of the heterogeneous impact of two shocks to the supply of mortgage credit: postcrisis regulatory burden and GSE financing cost changes. Small banks exploit regulation disproportionately affecting the largest four banks (Big4) and their ability to lend on balance sheet to strongly substitute for the retreating Big4. The erasure of guarantee fee (g-fee) discounts for large lenders facilitates small bank growth in GSE lending. Small banks also grow balance sheet loans in areas more exposed to g-fee hikes.
The Rise of Finance Companies and FinTech Lenders in Small Business Lending
Review of Financial Studies, 2022
Gopal, Manasa; Schnabl, Philipp
We document that finance companies and FinTech lenders increased lending to small businesses after the 2008 financial crisis. We show that most of the increase substituted for a reduction in bank lending. In counties in which banks had a larger market share before the crisis, finance companies and FinTech lenders increased their lending more. We find no effect of reduced bank lending on employment, wages, and new business creation by 2016. Our results suggest that finance companies and FinTech lenders are major suppliers of credit to small businesses and played an important role in the recovery from the 2008 financial crisis.
The Party Structure of Mutual Funds
Review of Financial Studies, 2022
Bubb, Ryan; Catan, Emiliano M.
We investigate the structure of mutual funds' corporate governance preferences as revealed by how they vote their shares in portfolio companies. We apply unsupervised learning tools from the machine learning literature to analyze mutual funds' votes and find that a parsimonious two-dimensional model can explain the bulk of mutual fund voting. The dimensions capture competing visions of corporate governance and are related to the leading proxy advisors' recommendations. Cluster analysis shows that mutual funds are organized into three "parties"--the Traditional Governance Party, Shareholder Reform Party, and Shareholder Protest Party--that follow distinctive philosophies of corporate governance and shareholders' role.
The Use and Misuse of Patent Data: Issues for Finance and Beyond
Review of Financial Studies, 2022
Lerner, Josh; Seru, Amit
Patents and citations are powerful tools increasingly used in financial economics (and management research more broadly) to understand innovation. Biases may result, however, from the interactions between the truncation of patents and citations and the changing composition of inventors. When aggregated at the firm level, these patent and citation biases can survive popular adjustment methods and are correlated with firm characteristics. These issues can lead to problematic inferences. We provide an actionable checklist to avoid biased inferences and also suggest machine learning as a potential new way to address these problems.
Regressive Mortgage Credit Redistribution in the Post-crisis Era
Review of Financial Studies, 2022
D'Acunto, Francesco; Rossi, Alberto G.
We document four secular trends about U.S. mortgage origination by traditional and FinTech lenders after the 2008-2009 financial crisis. First, since 2011, the overall number, size, and approval rate of small and medium-sized loans have been decreasing over time, relative to large loans. Second, the largest lenders redistribute their lending the most. Third, this loan-size redistribution of credit increases in the size of the lender. Fourth, the effects are stronger for mortgages further away from the conforming loan limit(s) in both directions. We argue that the supply of credit drives these secular trends, and we assess several potential economic mechanisms.
Fintech Borrowers: Lax Screening or Cream-Skimming?
Review of Financial Studies, 2021
Di Maggio, Marco; Yao, Vincent
We study the personal credit market using unique individual-level data covering fintech and traditional lenders. We show that fintech lenders acquire market share by lending first to higher-risk borrowers and then to safer borrowers, and rely mainly on hard information to make credit decisions. Fintech borrowers are significantly more likely to default than neighbor individuals with the same characteristics borrowing from traditional financial institutions. Furthermore, they tend to experience a short-lived reduction in the cost of credit, because their indebtedness increases more than non-fintech borrowers after loan origination. However, fintech lenders' pricing strategies are likely to take this into account.
Thousands of Alpha Tests
Review of Financial Studies, 2021
Giglio, Stefano; Liao, Yuan; Xiu, Dacheng
Data snooping is a major concern in empirical asset pricing. We develop a new framework to rigorously perform multiple hypothesis testing in linear asset pricing models, while limiting the occurrence of false positive results typically associated with data snooping. By exploiting a variety of machine learning techniques, our multiple-testing procedure is robust to omitted factors and missing data. We also prove its asymptotic validity when the number of tests is large relative to the sample size, as in many finance applications. To improve the finite sample performance, we also provide a wild-bootstrap procedure for inference and prove its validity in this setting. Finally, we illustrate the empirical relevance in the context of hedge fund performance evaluation.
Measuring Corporate Culture Using Machine Learning
Review of Financial Studies, 2021
Li, Kai; Mai, Feng; Shen, Rui; Yan, Xinyan
We create a culture dictionary using one of the latest machine learning techniques--the word embedding model--and 209,480 earnings call transcripts. We score the five corporate cultural values of innovation, integrity, quality, respect, and teamwork for 62,664 firm-year observations over the period 2001-2018. We show that an innovative culture is broader than the usual measures of corporate innovation--R&D expenses and the number of patents. Moreover, we show that corporate culture correlates with business outcomes, including operational efficiency, risk-taking, earnings management, executive compensation design, firm value, and deal making, and that the culture-performance link is more pronounced in bad times. Finally, we present suggestive evidence that corporate culture is shaped by major corporate events, such as mergers and acquisitions.
Selecting Directors Using Machine Learning
Review of Financial Studies, 2021
Erel, Isil; Stern, Lea H.; Tan, Chenhao; Weisbach, Michael S.
Can algorithms assist firms in their decisions on nominating corporate directors? Directors predicted by algorithms to perform poorly indeed do perform poorly compared to a realistic pool of candidates in out-of-sample tests. Predictably bad directors are more likely to be male, accumulate more directorships, and have larger networks than the directors the algorithm would recommend in their place. Companies with weaker governance structures are more likely to nominate them. Our results suggest that machine learning holds promise for understanding the process by which governance structures are chosen and has potential to help real-world firms improve their governance.
Microstructure in the Machine Age
Review of Financial Studies, 2021
Easley, David; Lopez de Prado, Marcos; O'Hara, Maureen; Zhang, Zhibai
Understanding modern market microstructure phenomena requires large amounts of data and advanced mathematical tools. We demonstrate how machine learning can be applied to microstructural research. We find that microstructure measures continue to provide insights into the price process in current complex markets. Some microstructure features with high explanatory power exhibit low predictive power, while others with less explanatory power have more predictive power. We find that some microstructure-based measures are useful for out-of-sample prediction of various market statistics, leading to questions about market efficiency. We also show how microstructure measures can have important cross-asset effects. Our results are derived using 87 liquid futures contracts across all asset classes.
Bond Risk Premiums with Machine Learning
Review of Financial Studies, 2021
Bianchi, Daniele; Buchner, Matthias; Tamoni, Andrea
We show that machine learning methods, in particular, extreme trees and neural networks (NNs), provide strong statistical evidence in favor of bond return predictability. NN forecasts based on macroeconomic and yield information translate into economic gains that are larger than those obtained using yields alone. Interestingly, the nature of unspanned factors changes along the yield curve: stock- and labor-market-related variables are more relevant for short-term maturities, whereas output and income variables matter more for longer maturities. Finally, NN forecasts correlate with proxies for time-varying risk aversion and uncertainty, lending support to models featuring both channels.
On the Rise of FinTechs: Credit Scoring Using Digital Footprints
Review of Financial Studies, 2020
Berg, Tobias; Burg, Valentin; Gombovic, Ana; Puri, Manju
We analyze the information content of a digital footprint--that is, information that users leave online simply by accessing or registering on a Web site--for predicting consumer default. We show that even simple, easily accessible variables from a digital footprint match the information content of credit bureau scores. A digital footprint complements rather than substitutes for credit bureau information and affects access to credit and reduces default rates. We discuss the implications for financial intermediaries' business models, access to credit for the unbanked, and the behavior of consumers, firms, and regulators in the digital sphere.
Empirical Asset Pricing via Machine Learning
Review of Financial Studies, 2020
Gu, Shihao; Kelly, Bryan; Xiu, Dacheng
We perform a comparative analysis of machine learning methods for the canonical problem of empirical asset pricing: measuring asset risk premiums. We demonstrate large economic gains to investors using machine learning forecasts, in some cases doubling the performance of leading regression-based strategies from the literature. We identify the best-performing methods (trees and neural networks) and trace their predictive gains to allowing nonlinear predictor interactions missed by other methods. All methods agree on the same set of dominant predictive signals, a set that includes variations on momentum, liquidity, and volatility.
How Valuable Is FinTech Innovation?
Review of Financial Studies, 2019
Chen, Mark A.; Wu, Qinxi; Yang, Baozhong
We provide large-scale evidence on the occurrence and value of FinTech innovation. Using data on patent filings from 2003 to 2017, we apply machine learning to identify and classify innovations by their underlying technologies. We find that most FinTech innovations yield substantial value to innovators, with blockchain being particularly valuable. For the overall financial sector, internet of things (IoT), robo-advising, and blockchain are the most valuable innovation types. Innovations affect financial industries more negatively when they involve disruptive technologies from nonfinancial startups, but market leaders that invest heavily in their own innovation can avoid much of the negative value effect.
To FinTech and Beyond
Review of Financial Studies, 2019
Goldstein, Itay; Jiang, Wei; Karolyi, G. Andrew
FinTech is about the introduction of new technologies into the financial sector, and it is now revolutionizing the financial industry. In 2017, when the academic finance community was not actively researching FinTech, the editorial team of the Review of Financial Studies launched a competition to develop research proposals focused on this topic. This special issue is the result. In this introductory article, we describe the recent FinTech phenomenon and the novel editorial protocol employed for this special issue following the Registered Reports format. We discuss what we learned from the submitted proposals about the field of FinTech and which ones we selected to be completed and ultimately come out in this special issue. We also provide several observations to help guide future research in the emerging area of FinTech.
The Role of Technology in Mortgage Lending
Review of Financial Studies, 2019
Fuster, Andreas; Plosser, Matthew; Schnabl, Philipp; Vickery, James
Technology-based ("FinTech") lenders increased their market share of U.S. mortgage lending from 2% to 8% from 2010 to 2016. Using loan-level data on mortgage applications and originations, we show that FinTech lenders process mortgage applications 20% faster than other lenders, controlling for observable characteristics. Faster processing does not come at the cost of higher defaults. FinTech lenders adjust supply more elastically than do other lenders in response to exogenous mortgage demand shocks. In areas with more FinTech lending, borrowers refinance more, especially when it is in their interest. We find no evidence that FinTech lenders target borrowers with low access to finance.