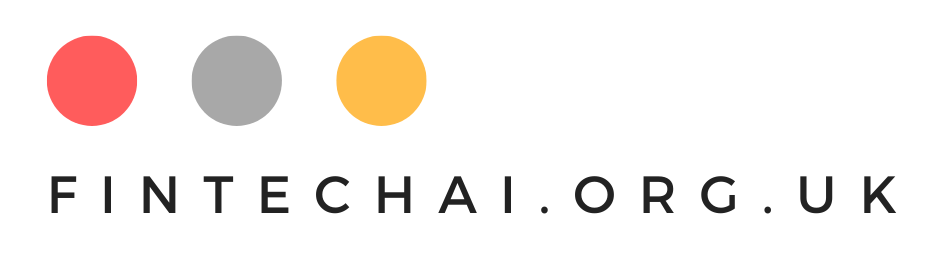
Infographics of Publications in Journal of Finance
Goal Setting and Saving in the FinTech Era
Journal of Finance, 2024
Gargano, Antonio; Rossi, Alberto G.
We study the effectiveness of saving goals in increasing individuals' savings using data from a Fintech app. Using a difference-in-differences identification strategy that randomly assigns users into a group of beta testers who can set goals and a group of users who cannot, we find that setting goals increases individuals' savings rate. The increased savings within the app do not reduce savings outside the app. Moreover, goal setting helps those individuals previously identified as having the lowest propensity to save. Matching App user survey responses to their behavior highlights the relative merits of monitoring and concreteness channels in explaining our findings.
Informed Trading Intensity
Journal of Finance, 2024
Bogousslavsky, Vincent; Fos, Vyacheslav; Muravyev, Dmitriy
We train a machine learning method on a class of informed trades to develop a new measure of informed trading, informed trading intensity (ITI). ITI increases before earnings, mergers and acquisitions, and news announcements, and has implications for return reversal and asset pricing. ITI is effective because it captures nonlinearities and interactions between informed trading, volume, and volatility. This data-driven approach can shed light on the economics of informed trading, including impatient informed trading, commonality in informed trading, and models of informed trading. Overall, learning from informed trading data can generate an effective informed trading measure.
Lender Automation and Racial Disparities in Credit Access
Journal of Finance, 2024
Howell, Sabrina T.; Kuchler, Theresa; Snitkof, David; Stroebel, Johannes; Wong, Jun
Process automation reduces racial disparities in credit access by enabling smaller loans, broadening banks' geographic reach, and removing human biases from decision making. We document these findings in the context of the Paycheck Protection Program (PPP), where private lenders faced no credit risk but decided which firms to serve. Black-owned firms obtained PPP loans primarily from automated fintech lenders, especially in areas with high racial animus. After traditional banks automated their loan processing procedures, their PPP lending to Black-owned firms increased. Our findings cannot be fully explained by racial differences in loan application behaviors, preexisting banking relationships, firm performance, or fraud rates.
The Virtue of Complexity in Return Prediction
Journal of Finance, 2024
Kelly, Bryan; Malamud, Semyon; Zhou, Kangying
Much of the extant literature predicts market returns with "simple" models that use only a few parameters. Contrary to conventional wisdom, we theoretically prove that simple models severely understate return predictability compared to "complex" models in which the number of parameters exceeds the number of observations. We empirically document the virtue of complexity in U.S. equity market return prediction. Our findings establish the rationale for modeling expected returns through machine learning.
Artificial Intelligence, Education, and Entrepreneurship
Journal of Finance, 2024
Gofman, Michael; Jin, Zhao
We document an unprecedented brain drain of Artificial Intelligence (AI) professors from universities from 2004 to 2018. We find that students from the affected universities establish fewer AI startups and raise less funding. The brain-drain effect is significant for tenured professors, professors from top universities, and deep-learning professors. Additional evidence suggests that unobserved city- and university-level shocks are unlikely to drive our results. We consider several economic channels for the findings. The most consistent explanation is that professors' departures reduce startup founders' AI knowledge, which we find is an important factor for successful startup formation and fundraising.
(Re-)imag(in)ing Price Trends
Journal of Finance, 2023
Jiang, Jingwen; Kelly, Bryan; Xiu, Dacheng
We reconsider trend-based predictability by employing flexible learning methods to identify price patterns that are highly predictive of returns, as opposed to testing predefined patterns like momentum or reversal. Our predictor data are stock-level price charts, allowing us to extract the most predictive price patterns using machine learning image analysis techniques. These patterns differ significantly from commonly analyzed trend signals, yield more accurate return predictions, enable more profitable investment strategies, and demonstrate robustness across specifications. Remarkably, they exhibit context independence, as short-term patterns perform well on longer time scales, and patterns learned from U.S. stocks prove effective in international markets.
Firm-Level Climate Change Exposure
Journal of Finance, 2023
Sautner, Zacharias; Van Lent, Laurence; Vilkov, Grigory; Zhang, Ruishen
We develop a method that identifies the attention paid by earnings call participants to firms' climate change exposures. The method adapts a machine learning keyword discovery algorithm and captures exposures related to opportunity, physical, and regulatory shocks associated with climate change. The measures are available for more than 10,000 firms from 34 countries between 2002 and 2020. We show that the measures are useful in predicting important real outcomes related to the net-zero transition, in particular, job creation in disruptive green technologies and green patenting, and that they contain information that is priced in options and equity markets.
Did FinTech Lenders Facilitate PPP Fraud?
Journal of Finance, 2023
Griffin, John M.; Kruger, Samuel; Mahajan, Prateek
In the $793 billion Paycheck Protection Program, we examine metrics related to potential misreporting including nonregistered businesses, multiple businesses at residential addresses, abnormally high implied compensation per employee, and large inconsistencies with jobs reported in another government program. These measures consistently concentrate in certain FinTech lenders and are cross-verified by seven additional measures. FinTech market share increased significantly over time, and suspicious lending by FinTechs in 2021 is four times the level at the start of the program. Suspicious loans are being overwhelmingly forgiven at rates similar to other loans.
Biased Auctioneers
Journal of Finance, 2023
Aubry, Mathieu; Kraussl, Roman; Manso, Gustavo; Spaenjers, Christophe
We construct a neural network algorithm that generates price predictions for art at auction, relying on both visual and nonvisual object characteristics. We find that higher automated valuations relative to auction house presale estimates are associated with substantially higher price-to-estimate ratios and lower buy-in rates, pointing to estimates' informational inefficiency. The relative contribution of machine learning is higher for artists with less dispersed and lower average prices. Furthermore, we show that auctioneers' prediction errors are persistent both at the artist and at the auction house level, and hence directly predictable themselves using information on past errors.
Do Municipal Bond Dealers Give Their Customers 'Fair and Reasonable' Pricing?
Journal of Finance, 2023
Griffin, John M.; Hirschey, Nicholas; Kruger, Samuel
Municipal bonds exhibit considerable retail pricing variation, even for same-size trades of the same bond on the same day, and even from the same dealer. Markups vary widely across dealers. Trading strongly clusters on eighth price increments, and clustered trades exhibit higher markups. Yields are often lowered to just above salient numbers. Machine learning estimates exploiting the richness of the data show that dealers that use strategic pricing have systematically higher markups. Recent Municipal Securities Rulemaking Board rules have had only a limited impact on markups. While a subset of dealers focus on best execution, many dealers appear focused on opportunistic pricing.
Attention-Induced Trading and Returns: Evidence from Robinhood Users
Journal of Finance, 2022
Barber, Brad M.; Huang, Xing; Odean, Terrance; Schwarz, Christopher
We study the influence of financial innovation by fintech brokerages on individual investors' trading and stock prices. Using data from Robinhood, we find that Robinhood investors engage in more attention-induced trading than other retail investors. For example, Robinhood outages disproportionately reduce trading in high-attention stocks. While this evidence is consistent with Robinhood attracting relatively inexperienced investors, we show that it is also driven in part by the app's unique features. Consistent with models of attention-induced trading, intense buying by Robinhood users forecasts negative returns. Average 20-day abnormal returns are -4.7% for the top stocks purchased each day.
Anomalies and the Expected Market Return
Journal of Finance, 2022
Dong, Xi; Li, Yan; Rapach, David E.; Zhou, Guofu
We provide the first systematic evidence on the link between long-short anomaly portfolio returns--a cornerstone of the cross-sectional literature--and the time-series predictability of the aggregate market excess return. Using 100 representative anomalies from the literature, we employ a variety of shrinkage techniques (including machine learning, forecast combination, and dimension reduction) to efficiently extract predictive signals in a high-dimensional setting. We find that long-short anomaly portfolio returns evince statistically and economically significant out-of-sample predictive ability for the market excess return. The predictive ability of anomaly portfolio returns appears to stem from asymmetric limits of arbitrage and overpricing correction persistence.
Predictably Unequal? The Effects of Machine Learning on Credit Markets
Journal of Finance, 2022
Fuster, Andreas; Goldsmith-Pinkham, Paul; Ramadorai, Tarun; Walther, Ansgar
Innovations in statistical technology in functions including credit-screening have raised concerns about distributional impacts across categories such as race. Theoretically, distributional effects of better statistical technology can come from greater flexibility to uncover structural relationships or from triangulation of otherwise excluded characteristics. Using data on U.S. mortgages, we predict default using traditional and machine learning models. We find that Black and Hispanic borrowers are disproportionately less likely to gain from the introduction of machine learning. In a simple equilibrium credit market model, machine learning increases disparity in rates between and within groups, with these changes attributable primarily to greater flexibility.