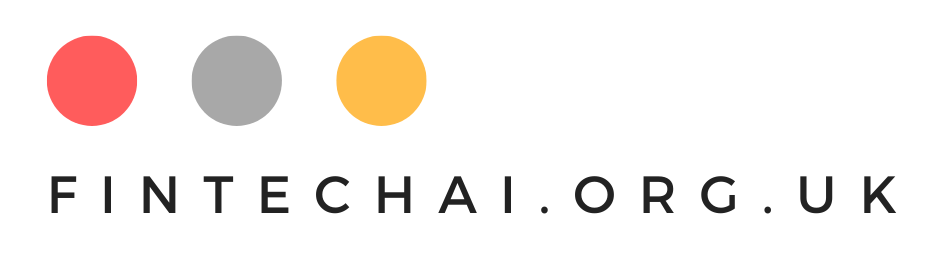
Key Findings
Superior Performance of CNN-Based Image Analysis
Image-based CNN predictions outperform traditional trend signals with annualized Sharpe ratios up to 7.2 for equal-weight portfolios
Successful International Transfer Learning
CNN models trained on U.S. data transfer effectively to international markets, outperforming locally trained models in 21 out of 26 countries
Robust Time-Scale Transfer
Models trained on high-frequency data successfully predict returns at lower frequencies, demonstrating the fractal nature of price patterns
Weekly Strategy Performance Comparison
- CNN strategies achieve Sharpe ratios of 7.2, 6.8, and 4.9 for 5-, 20-, and 60-day images respectively
- Traditional strategies like WSTR and TREND achieve lower Sharpe ratios of 2.8 and 2.9
- CNN strategies maintain superior performance even in value-weighted portfolios
International Transfer Learning Success
- Transfer learning improves performance in 80% of international markets
- Average Sharpe ratio increases from 2.3 (local training) to 3.6 (transfer) for equal-weight portfolios
- Benefits are particularly strong for markets with fewer stocks
Time-Scale Transfer Performance
- Time-scale transfer achieves 2.1 Sharpe ratio for 20-day forecasts
- Performance comparable to direct training (2.2 Sharpe ratio)
- Demonstrates pattern self-similarity across time scales
Contribution and Implications
- Introduces novel approach combining CNN with price chart images for return prediction
- Demonstrates the universal nature of price patterns across markets and time scales
- Provides practical framework for applying deep learning to technical analysis
Data Sources
- Weekly strategy performance chart based on Table I performance metrics
- International transfer results derived from Table X comparing local vs transfer performance
- Time-scale transfer visualization constructed using data from Table XI equal-weight portfolio results