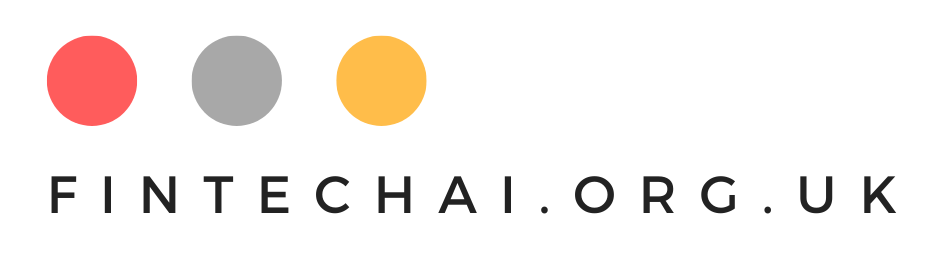
Key Findings
Autonomous Price Collusion
Q-learning pricing algorithms systematically learn to collude and charge supracompetitive prices, achieving 70-90% of monopoly profits without any explicit communication or instruction.
Punishment Strategies
Algorithms learn sophisticated punishment strategies with finite duration and gradual return to pre-deviation prices, making price cuts unprofitable in over 95% of cases.
Robust Collusion
Collusive behavior persists across different market conditions including asymmetric costs, stochastic demand, and varying numbers of competitors.
Profit Gains Across Market Conditions
- Baseline duopoly achieves 85% of monopoly profits
- Three-firm markets still maintain 64% profit gains
- Four-firm markets achieve 56% of monopoly profits
Cost Asymmetry Effects
- Collusion persists even with significant cost differences between firms
- Profit gains remain above 70% even with 75% cost differential
- Less efficient firms receive disproportionately higher profit shares
Price Response After Deviation
- Initial punishment phase with price war
- Gradual return to pre-deviation prices over 5-7 periods
- Deviation reduces cheater's profits by 3-4% on average
Contribution and Implications
- First demonstration that AI pricing algorithms can autonomously learn to collude without communication
- Suggests need to reconsider antitrust policy approaches as algorithmic pricing becomes more prevalent
- Opens possibility for new forms of antitrust intervention through direct testing of pricing algorithms
Data Sources
- Profit gains chart based on results reported in Section V.A on number of players
- Cost asymmetry effects visualized from Table 4 data on varying cost differentials
- Price deviation responses constructed from data in Tables 2-3 and Figure 4