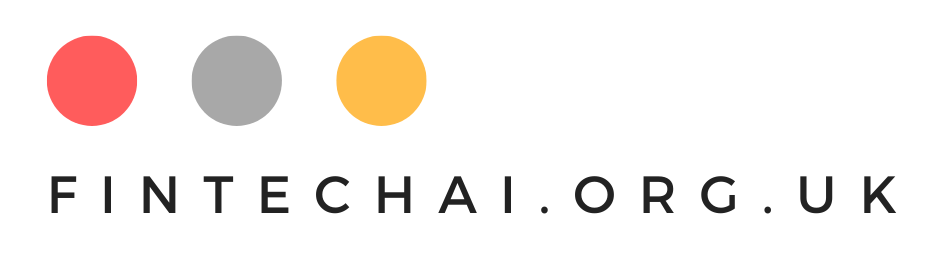
Key Findings
Large Price Distortion
Asymmetric information leads to equilibrium interest rates that are 22 percentage points higher than efficient levels (30.4% vs 8.5%)
Small Welfare Loss
Despite high price distortion, overall welfare loss is only 0.8% of loan amount (~¥50 or $7.20 per applicant) due to inelastic demand
Credit Score Impact
Welfare losses are 4x larger for low credit score borrowers (1.7%) compared to high credit score borrowers (0.4%)
Interest Rate Effects on Take-up and Charge-offs
- Low-Price group (21.5% interest) had 65% take-up rate vs 58% for High-Price group (36% interest)
- High-Price group had 13% charge-off rate vs 11% for Low-Price group
- Results indicate presence of asymmetric information - higher rates attract riskier borrowers
Equilibrium vs Efficient Outcomes
- Competitive equilibrium interest rate (30.4%) is substantially higher than efficient rate (8.5%)
- Despite large price difference, equilibrium quantity (60.8%) is only 10 percentage points lower than efficient quantity (70.3%)
- Small quantity effect due to inelastic borrower demand (elasticity of -0.13)
Credit Score Heterogeneity
- Welfare losses are 1.7% of loan amount for low credit score borrowers (Rating 2-4)
- Welfare losses are only 0.4% for high credit score borrowers (Rating 1)
- Interest rate sensitivity of charge-offs is 2x higher for low credit score borrowers
Contribution and Implications
- First study to quantify welfare costs of asymmetric information in fintech lending market
- Develops methodology to measure welfare losses in credit markets that can be applied broadly
- Small welfare losses suggest limited role for policy interventions like interest rate subsidies or loan guarantees
- Results specific to Chinese fintech market - welfare costs could differ in other credit markets
Data Sources
- Take-up and charge-off rates from Figure 2 showing experimental results across treatment groups
- Equilibrium and efficient outcomes calculated from demand and cost estimates in Table 2
- Credit score heterogeneity analysis based on Table 3 comparing outcomes across rating categories