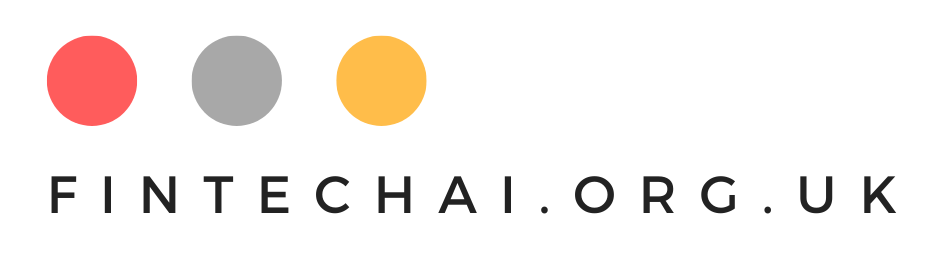
Key Findings
Nearest Neighbor Matching Efficiency
When the number of nearest neighbors (M) is allowed to diverge with sample size, the matching estimator achieves semiparametric efficiency and double robustness for estimating average treatment effects.
Computational Advantage
The proposed nearest neighbor matching method provides computationally efficient estimation with subquadratic time complexity while maintaining statistical optimality.
Bias Correction Performance
Bias-corrected matching estimators demonstrate strong empirical performance across different sample sizes and matching specifications, with coverage rates close to nominal levels.
Treatment Effect Estimation Performance
- Root Mean Squared Error (RMSE) decreases consistently as sample size increases
- Performance improves with larger sample sizes across all matching specifications
- Diverging M shows better performance compared to fixed M values
Coverage Rate Performance
- 95% coverage rates approach nominal level as sample size increases
- Both SE and AISE methods provide reliable confidence intervals
- Coverage performance is robust across different M specifications
Computational Efficiency Analysis
- Bias decreases with increasing sample size
- Diverging M specifications show minimal bias
- Computational efficiency maintained with larger samples
Contribution and Implications
- Provides theoretical justification for using diverging number of matches in nearest neighbor matching
- Demonstrates computational efficiency while maintaining statistical optimality
- Bridges gap between matching methods and modern machine learning approaches
- Offers practical guidance for implementing matching estimators in large-scale studies
Data Sources
- RMSE Chart: Constructed using data from Table I of the article, showing root-mean-squared-error values for different sample sizes and matching specifications
- Coverage Rate Chart: Based on Table I's 95% coverage rates for both SE and AISE methods
- Bias Chart: Derived from the bias columns in Table I, showing absolute bias values across sample sizes