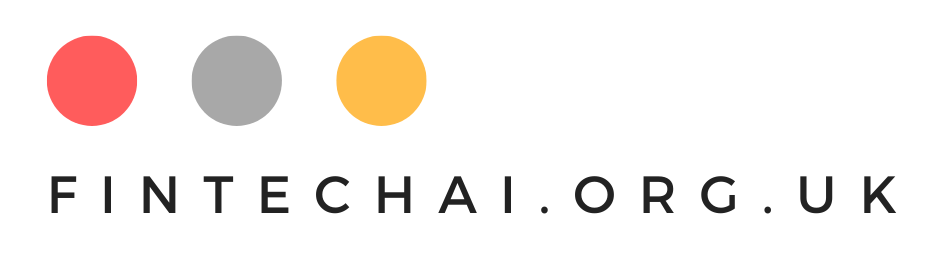
Key Findings
Valid Bootstrap Method for Cube Root Asymptotics
Developed a new bootstrap-based distributional approximation method that restores consistency by modifying the shape of the criterion function
Performance Comparison
The proposed method achieves better coverage rates and shorter confidence intervals compared to standard bootstrap and m-out-of-n bootstrap methods
Wide Applicability
Method works effectively across multiple econometric estimators including maximum score, panel data, and machine learning applications
Coverage Rates Across Bootstrap Methods
- Standard bootstrap shows poor coverage (~64%)
- M-out-of-n bootstrap achieves better coverage but with wider intervals
- Proposed method maintains close to 95% coverage rate
Confidence Interval Lengths
- M-out-of-n bootstrap produces wider confidence intervals
- Proposed method achieves shorter interval lengths while maintaining coverage
- Results consistent across different simulation designs (DGP 1-3)
Method Performance Across Estimators
- Successfully applied to maximum score estimation
- Effective for panel data models
- Applicable to machine learning classification problems
Contribution and Implications
- First valid bootstrap-based method for cube root asymptotics that modifies objective function rather than sampling distribution
- Provides practical inference tool for important econometric estimators previously lacking valid bootstrap procedures
- Computationally simple implementation requiring only estimation of Hessian matrix
Data Sources
- Coverage rates and confidence interval lengths based on Table I simulation results
- Performance comparison charts constructed using data from Monte Carlo experiments with n=1000, B=2000 bootstrap replications
- Method applications data derived from empirical examples in Sections 4.1-4.4